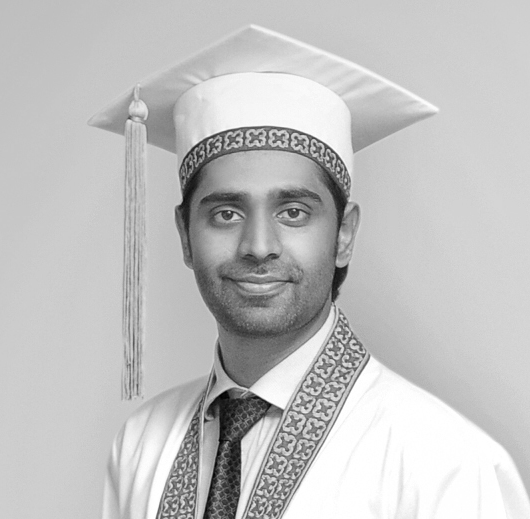
MUHAMMAD MURSALIN LARIK
Aspiration Statement
I want to pursue my career after my undergraduate studies as an entrepreneur. I am interested in conducting research in robotics and computer vision. After some years of graduation, I will apply to top graduate schools in the United States and Europe to gain exposure to research.
Core Skills
- Machine Learning
- Computer Vision
- Python
- MATLAB
- Robotics
- Geographic Information Systems (GIS)
- Field-Programmable Gate Arrays (FPGA)
- A/B Testing
Academic Awards / Achievements
- Université de Lorraine - Master's degree GENIAL
- Dean’s List - Spring 2017 & Spring 2018
- High Leap Scholarship (Spring 2017)
- Stanford University International Honors Program (Summer 2018)
- Global UGrad at Florida State University (Spring 2019)
Experience
Leadership / Meta-curricular
- Attended Florida International Leadership Conference 2019
- Govt. of Sindh, Pakistan Disaster & Humanitarian Relief - Volunteer
Internship / Volunteer Work
- Afiniti - Data Scientist AI Production (Mar 2021 - Present)
- Shape of Water (start-up) - Co-founder
- United Energy Pakistan Limited - Intern
- Habib University - Teaching Assistant
- Fuchsia Magazine - Marketing Assistant
Publications / Creative Projects
- Study of effect of guard zones in ad-hoc networks, solving hand-drawn circuits using deep leaning and image processing.
- Research in ad hoc network and stochastic geometry. Paper on the effect of guard zones and process of thinning the nodes on the performance measures in an ad hoc network.
Final Year Project
Project Title
Shape of Water (Multidisciplinary Project EE and SDP)
Description
Karachi, being Pakistan’s largest city, is the sixth most water-stressed city in the world. Our research showed that coupled with water shortages is a severe lack of management of existing urban water resources, due to a lack of planning and updated data on underground pipeline supply infrastructure. Around 42% of the extracted water gets lost or stolen. Leveraging the Ground Penetrating Radar (GPR) for utility detection, the project addresses automation of data interpretation of GPR’s radargrams through Machine Learning for the purpose of implementing the technology on a mass scale throughout the city. This project will compare the results of Logistic Regression for pipe detection with Deep Convolutional Neural Networks (MobileNet, ResNet-50 and Inception-Net). Additionally, hyperbola localization for depth estimation and pipe localization was done through Digital Image Processing and Yolo. (Group Project-Interdisciplinary)